The artificial intelligence (AI Testing) field has made incredible strides, leading to applications that assist in daily tasks, business operations, and complex problem-solving.
However, as AI becomes more embedded in society, the issue of bias within these systems has come to light. The presence of bias in AI can have serious consequences. The results generated by AI systems can influence decisions that affect people’s lives.
The identification of bias within AI is a complex and ongoing challenge, and this article will explore how testing can help, the methods used, and what it means for the future of AI.
What is Bias in AI Systems?
The AI systems learn from the data they are trained on. If this data contains patterns of bias, the system may replicate them, leading to results that are skewed or discriminatory.
Bias in AI can affect decisions ranging from hiring to loan approvals and even criminal justice. It is crucial to understand and mitigate this to ensure fairness.
- Bias can lead to unfair treatment.
- It can affect important decisions in life.
- Identifying bias is necessary to improve AI.
Types Of Bias Found in AI Systems
The algorithmic bias occurs when the rules or models used by AI favor one group over another, sometimes unintentionally. For instance, if an AI system is trained to identify qualified candidates for a job but was built on biased data, it might favor certain demographics over others.
The data bias is embedded when training datasets reflect historical prejudices. If a system learns from biased data, it will replicate those biases. This is why testing AI for bias is crucial before deploying it in real-world applications.
Can AI Testing Effectively Identify Bias?
The effectiveness of AI testing to identify bias depends on various factors. It involves evaluating how the AI system makes decisions and checking if certain groups are treated unfairly. This process requires careful analysis and the use of specialized tools designed to detect patterns of bias.
Bias in AI can have different impacts depending on the industry. In healthcare, biased AI can lead to incorrect diagnoses. In finance, it can result in unfair loan decisions. AI testing helps ensure that these systems perform fairly across different sectors.
Why is identifying Bias Important in AI Testing?
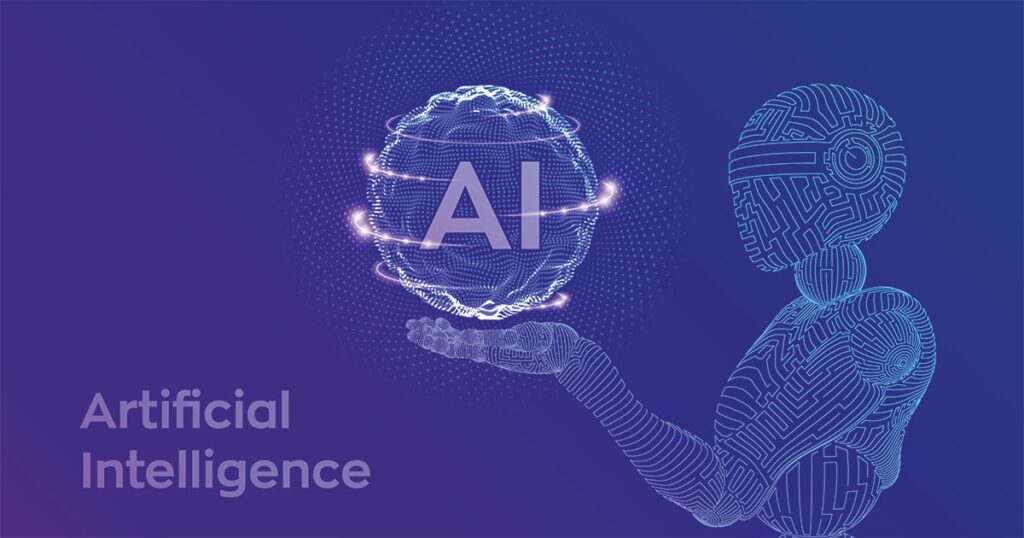
The identifying bias in AI systems is critical to ensure fairness and accuracy. If bias is not addressed, it can lead to serious ethical and legal consequences. Identifying bias in AI testing is crucial because it ensures fairness, accuracy, and reliability in AI systems.
- Bias can lead to unfair outcomes, favoring certain groups while disadvantaging others.
- Addressing bias also builds trust in AI systems, as users are more likely to trust and adopt technologies that are fair.
It is important to detect bias early in the testing process to prevent discriminatory practices and ensure that the AI models work correctly for everyone, regardless of their background or identity.
Challenges in Detecting Bias in AI Systems
The challenges include understanding the complexity of AI algorithms. The bias might not be immediately visible, and advanced tools are often required to analyze data and algorithms deeply. Moreover, AI systems can sometimes learn biases that were not evident during development.
How Do Biases Enter AI Systems?
Biases can enter at various stages
- During data collection if the data is not diverse.
- When developers unintentionally incorporate their own biases into the model.
- If the algorithms themselves have flaws.
Understanding how biases enter can help in creating strategies to avoid them.
Common Tools Used For Identifying Bias in AI
Several tools and techniques have been developed to help detect bias
- Fairness Indicators: A tool that helps visualize potential biases.
- AI Fairness 360: IBM’s toolkit that detects and mitigates bias.
- LIME (Local Interpretable Model-agnostic Explanations): Helps to understand the behavior of AI models and find hidden biases.
Best Practices For Reducing Bias in AI
The reducing bias in AI systems requires a structured approach
- Use diverse and balanced datasets.
- Regularly audit and update algorithms.
- Include multiple stakeholders during development to identify potential biases.
- Test thoroughly before deploying AI models.
The Role Of Diverse Data in AI Testing
If an AI is trained only on data from one demographic, it will not perform well for others. This is where bias often starts, making it vital to use balanced datasets. The role of diverse data in AI testing is crucial for creating reliable and unbiased models.
It ensures that AI systems can perform well across different scenarios and populations. Without diverse data, AI models may fail to recognize or misinterpret certain inputs, leading to errors and unfair outcomes. Diverse data helps in training AI to handle various languages, cultures, and situations, improving accuracy and fairness.
Examples Of AI Bias in Real-World Applications
The AI has been found to show bias in several real-world scenarios
- Hiring Processes: AI systems favoring certain genders.
- Facial Recognition: Difficulty recognizing darker skin tones.
- Healthcare: Biases leading to inaccurate treatment suggestions.
These examples highlight the importance of testing for bias before deploying AI.
Ethical Concerns Around AI Bias
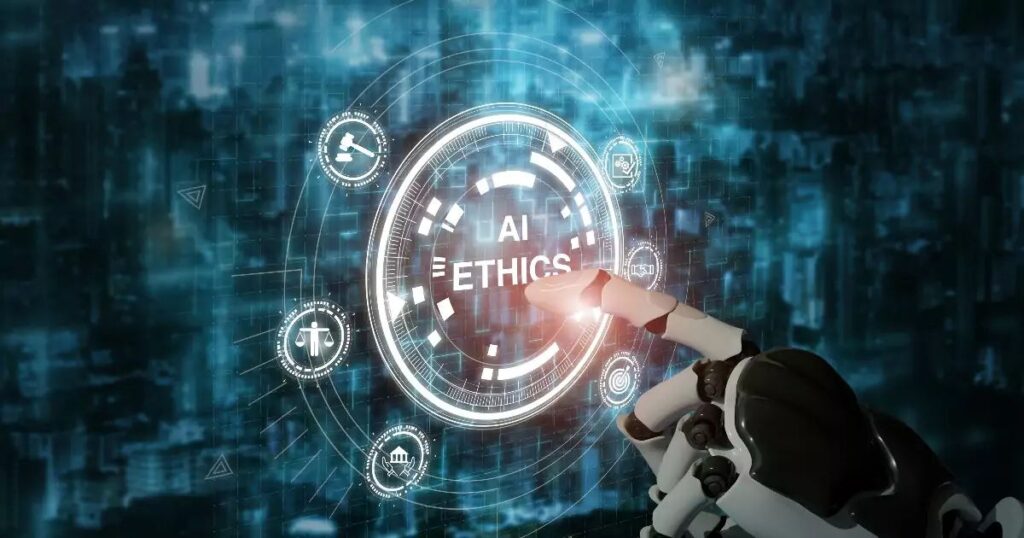
The ethical implications of biased AI can be severe. The systems can reinforce stereotypes and inequalities. Ensuring that AI is fair and unbiased is not just a technical issue but a moral one. Developers must be conscious of the broader impact of their systems.
Techniques To Test For Bias in AI
Several techniques can be employed
- Data Analysis: Check the data for diversity and fairness.
- Algorithm Auditing: Regular audits of algorithms can help spot biases.
- Outcome Testing: Ensure that the outcomes are fair across different groups.
What Are The Limitations Of Bias Testing in AI?
The bias testing can reveal many issues, but it has its limitations. The detection tools are only as good as the data they are provided. Also, biases can be subtle and hard to detect, especially if they are deeply embedded in the algorithms.
Future Innovations in AI Bias Testing
The future of AI bias testing looks promising. New tools are being developed that use advanced analytics to spot even the most subtle biases. There is also a push towards making AI models more transparent, which will make bias testing easier.
Case Studies Companies Addressing AI Bias
Several companies are taking steps to address AI bias
- Google: Introduced fairness indicators in their AI tools.
- Microsoft: Working on responsible AI initiatives.
- IBM: Launched AI Fairness 360 toolkit.
These companies show that addressing bias is possible with the right approach.
Moving Towards Fair And Transparent AI
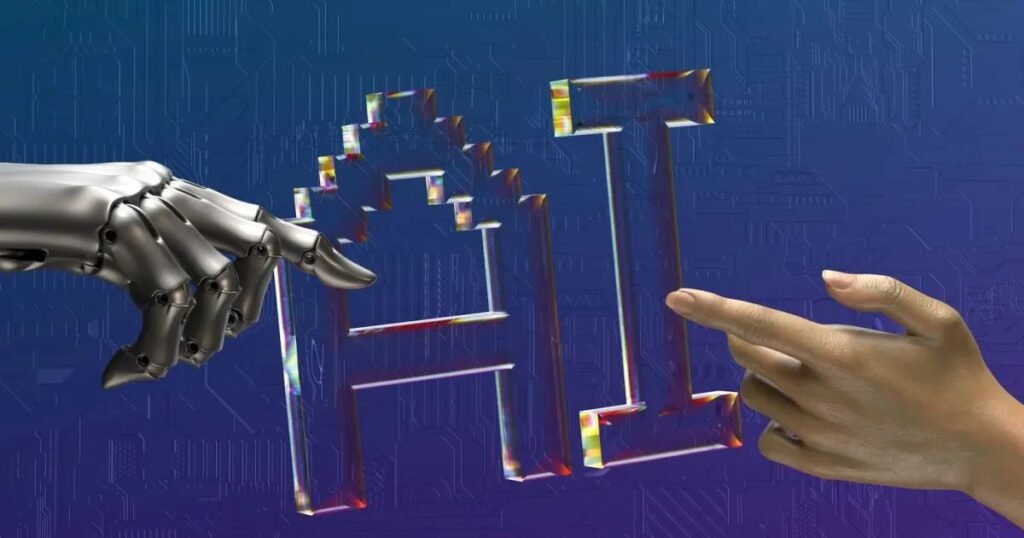
The future of AI should be one where systems operate without bias. This requires a joint effort from developers, researchers, and companies. Regular testing and ethical considerations can help build fairer systems. The development of fair and transparent AI has become a critical focus in the tech world.
As AI systems grow more influential in decision-making processes, ensuring they are unbiased and transparent is essential for building trust. It requires clear algorithms, open data usage, and accountability in how AI impacts people.
Frequently Asked Questions
Can AI testing fully remove bias from a system?
The testing can significantly reduce bias, but it may not eliminate it completely. Continuous monitoring is necessary.
What is the role of data in AI bias?
The data is crucial. If the data is biased, the AI system will learn and replicate those biases. Ensuring diverse and balanced datasets is key.
How can companies address bias in their AI systems?
The companies can implement regular audits, use bias detection tools, and ensure diverse teams are involved in the development process.
Are there any regulations around AI bias?
The regulations are being developed in various regions, focusing on transparency and fairness to ensure ethical AI usage.
Conclusion
AI testing identify bias is a crucial one as AI continues to integrate into various sectors. The answer is yes, but it requires careful analysis, tools, and ongoing vigilance.
AI testing can highlight biases and help in mitigating them, but developers must also remain conscious of the ethical implications. By following best practices, using diverse datasets, and implementing robust testing, it is possible to build fairer and more reliable AI systems.